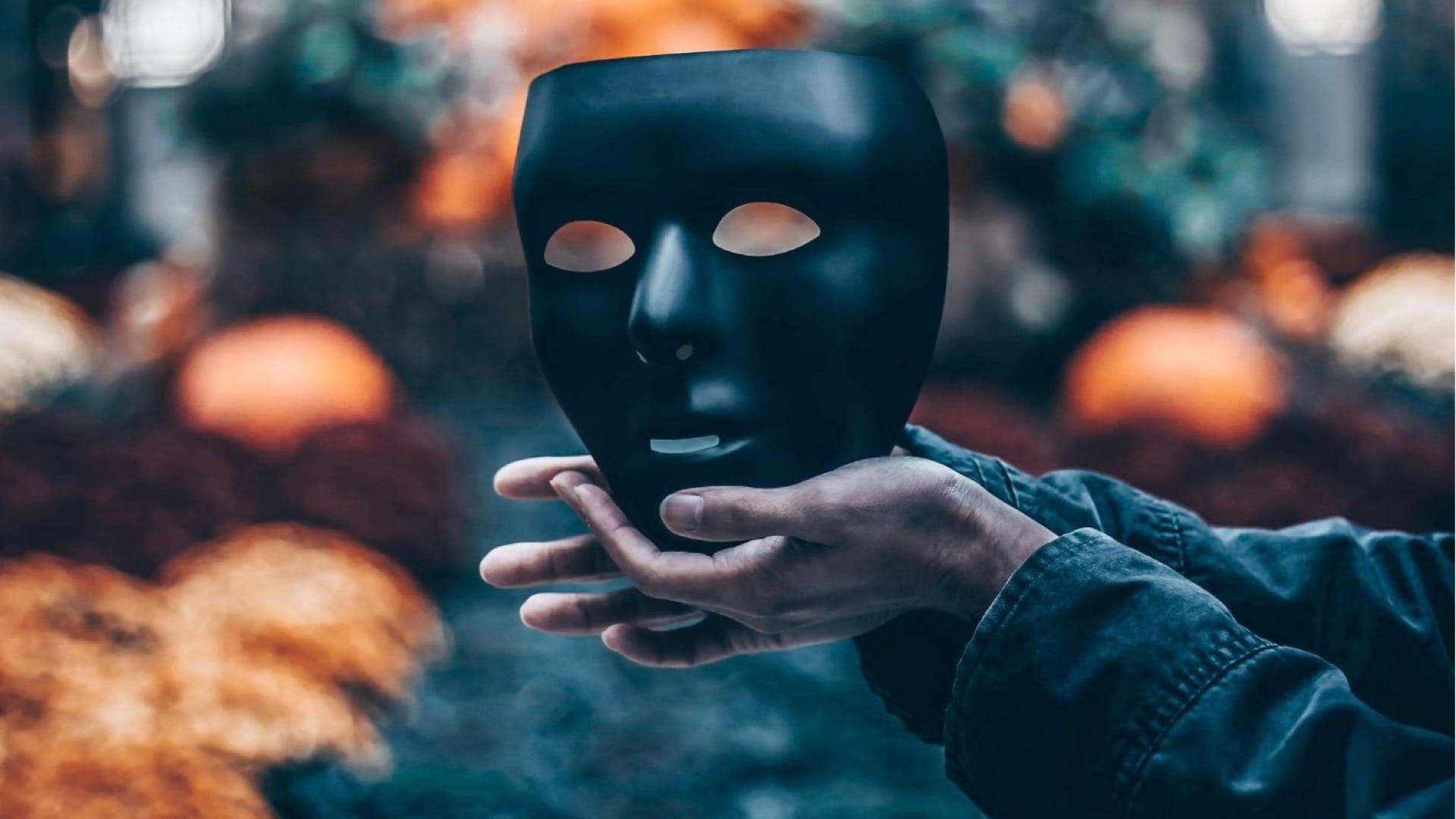
Anonymous vs. Anonymized: learn the difference
Understanding Anonymity in Sensor Data: discover the inherent privacy characteristics of each type of Sensor data and the potential risks associated with anonymizing sensitive information
Anonymous: This term denotes something that originates without a known or discernible identity, either from the start or intentionally kept hidden.
Anonymized: This term describes the characteristic of an object that, while once containing identifiable information, has undergone a process to remove or alter that information, rendering it non-traceable to an individual.
So, if you were to refer to computer perception data:
- Anonymous data: Data that never had identifiable markers to begin with.
- Anonymized data: Data that once contained identifiable markers but has since been processed to remove or alter them for privacy reasons.
Data Capture: Cameras vs. LiDAR and Radar
Curious about how these sensors compare? Check out our related article:
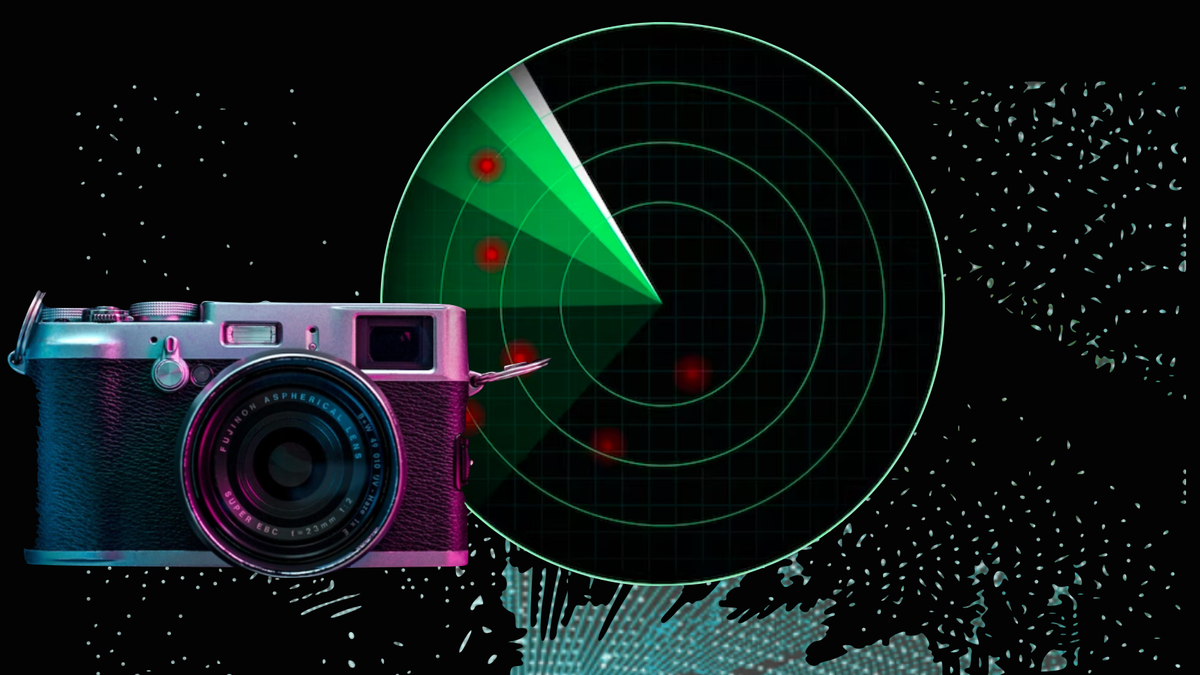
Cameras, whether traditional monocular types or stereo-vision (often referred to as 3D cameras), capture a wealth of detail from their field of view. This can include the minute facial features, clothing patterns, and sometimes even unique postures or gestures of individuals. Thus, the data from cameras inherently contains identifiable information about people present in their line of sight.
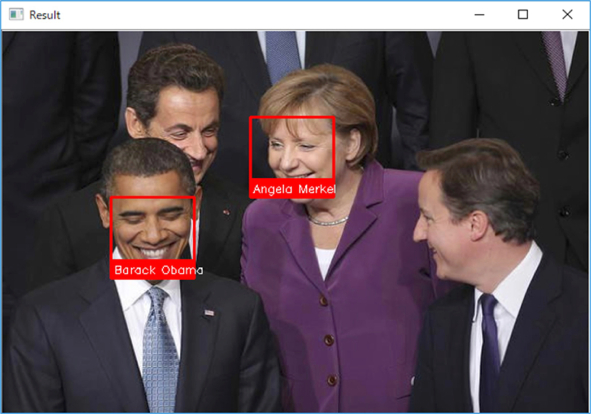
On the other hand, sensors like LiDAR and Radar work differently. They gauge distances by emitting laser pulses or radio waves and calculating the time they take to bounce back after hitting an object.
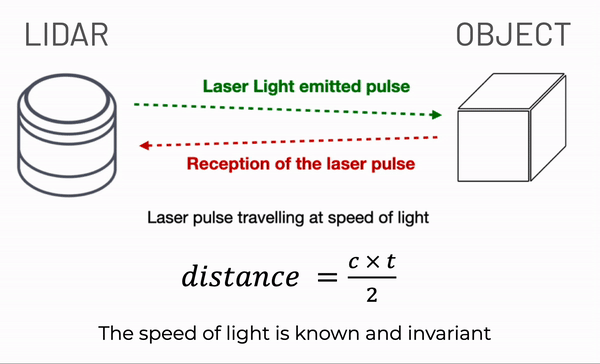
Consequently, these sensors capture general outlines and shapes without the intricate details that can be used to identify an individual.
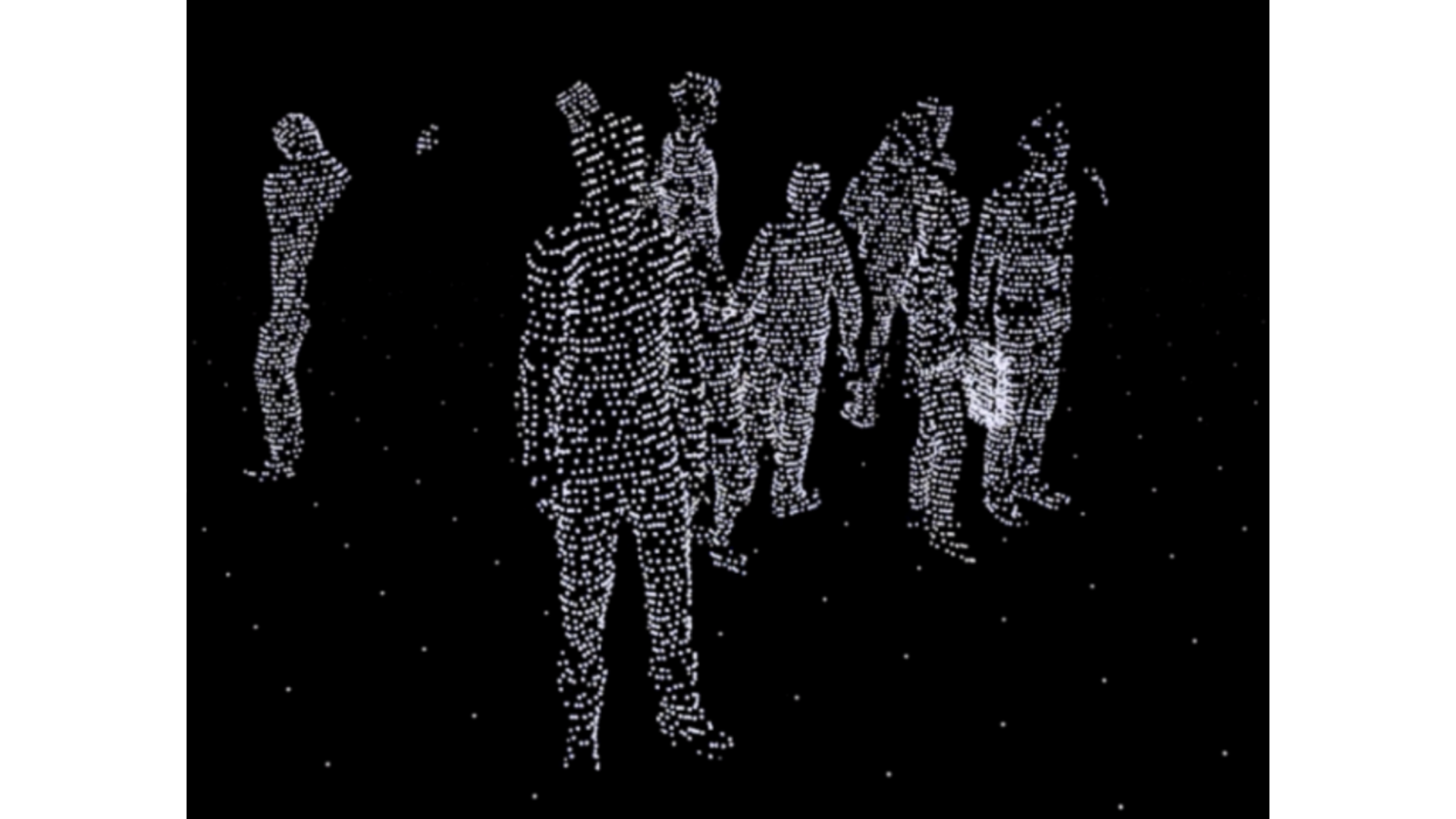
This leads us to conclude:
- LiDAR or Radar data is anonymous by nature.
- Camera-based images are not anonymous. However, they can attain an 'anonymized' status if they undergo a meticulous process designed to strip away identifiable data.
The Risks of Anonymizing Data
While it's commendable to convert inherently identifiable data into an anonymized form, this process isn't without its risks. The fundamental problem lies in the transition period – from the moment data is captured to its eventual anonymization.
For instance, if a system captures a person's image, and the goal is to anonymize it, even if processing is done in real-time, there's a time frame, however brief, when the raw, identifiable image exists. During this period, software programs reading the data (even if it's temporarily stored in volatile memory) have access to the original, non-anonymized information.
One might argue that these risks can be minimized using Edge AI processing, where data is processed directly on the capturing device or sensor.
While this approach does limit the exposure of raw data, it doesn't eliminate the risk entirely. There's still a brief moment when the original data exists before being processed, representing a potential vulnerability.
In Conclusion
As technology continues to advance, the conversation around data privacy becomes even more critical. Understanding the inherent nature of the data our devices collect is paramount.
When leveraging camera-based images is the only option, anonymizing the captured data certainly moves us closer to safeguarding user privacy. However, it's vital to be cognizant of the intrinsic risks that come with it.
As we navigate this space, our aim should always be to adopt robust solutions that place user privacy at the forefront. This includes exploring 3D Perception solutions like those based on LiDAR technology, which, beyond ensuring privacy, offer a plethora of other benefits:
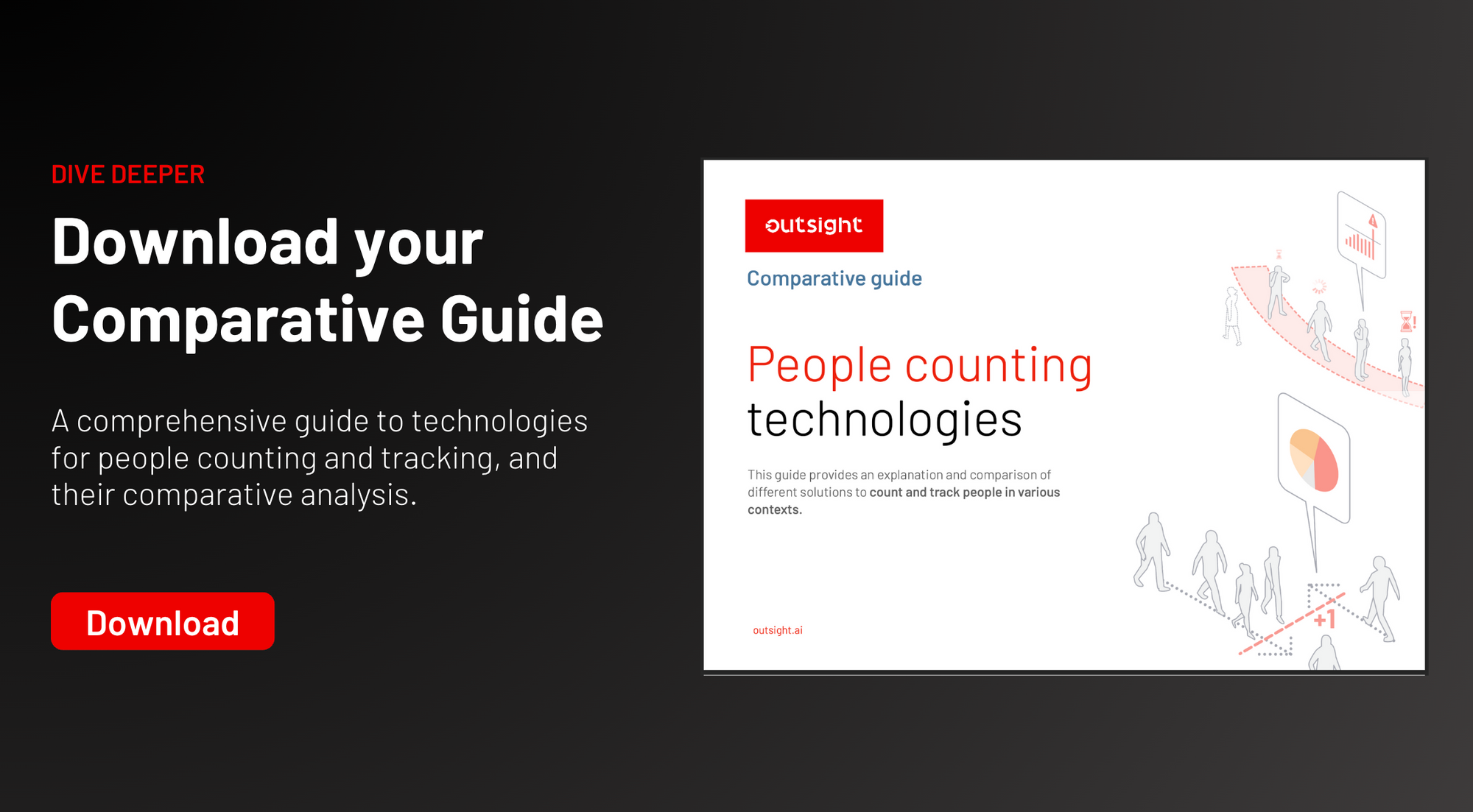